Insights
3 Ways to Drive eCommerce Growth With AI
AI can deliver a wealth of benefits for your eCommerce business.
- Article
- 3 MIN READ
- Nov 2, 2022
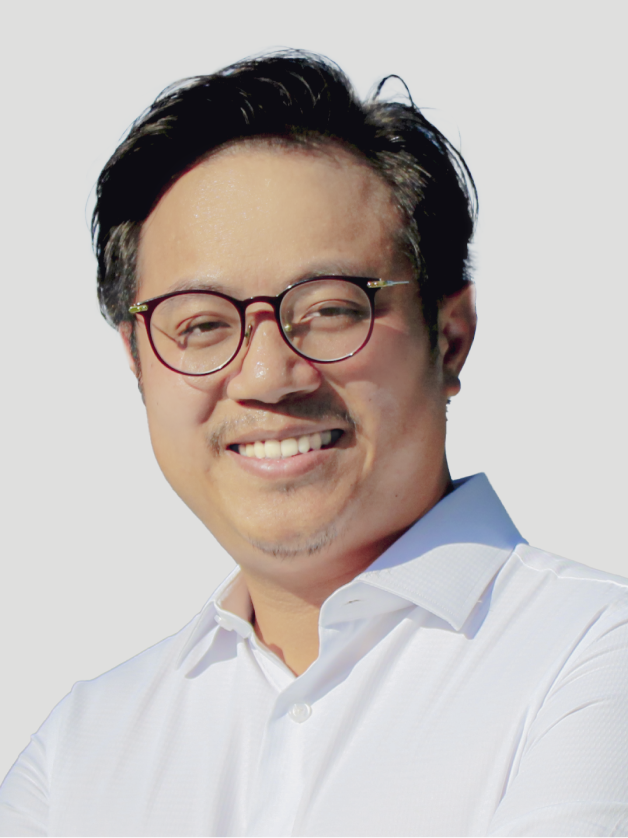
Head of Data
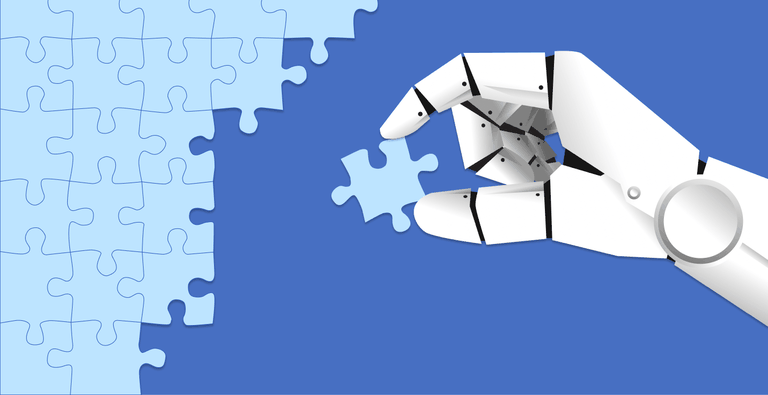
Summary
Here’s a staggering set of statistics for you.
Over the last four years, the number of businesses adopting artificial intelligence grew by 270%. And by 2025, it’s estimated that AI will manage 95% of all customer interactions through eCommerce stores.
This rapid acceleration of AI-supported eCommerce is redefining the digital landscape and driving many businesses to innovate in order to remain competitive. Now, more than ever, the company offering the best product recommendations and the smoothest checkout process for customers ends up miles ahead.
But integrating AI into your business is no small feat. You need the right foundations in place, the right team, and clear goals on how you’ll measure success. On top of this, it’s easy to get distracted. While AI has the power to unlock massive growth, integrating it into your world should not be the end goal. Rather, improving customer experiences that drive business growth is the key.
At Apply Digital, we’ve worked with many eCommerce companies that want to start using AI for the first time, or are looking to expand their current AI capabilities. We’ve seen what works, and what’s a massive waste of time. Without the right knowledge and guidance, you’re more likely to fall prey to shiny object syndrome and invest in technology that won’t move the needle toward your goals.
Below are three elements to consider when evaluating how to add AI to your tech ecosystem.
1. Set proper foundations
Before you get started with any AI tools, it’s helpful to lay some foundations.
First, it’s useful to understand the difference between AI and machine learning tools. AI is the general ability of your program to think, reason, and make decisions in behavior patterns that mimic human intelligence. Machine learning (ML), on the other hand, is the set of technologies and algorithms that learn and improve predictions over time. Great machine learning enables AI.
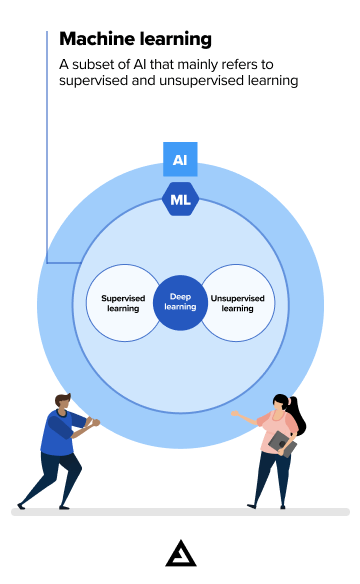
One of the biggest mistakes we see leaders make is jumping into building AI or machine learning solutions without understanding this difference, or neglecting to clearly establish the business problem they’re trying to solve. Before spending money on a tool, you need a firm grasp on what you want to optimize for and how you’ll measure success.
For example, maybe you want to increase conversions on your sales page by 5%. You could use many tactics to achieve this, and AI is certainly one of them. By quantifying this goal from the start, it will be easier to identify which AI tool you need. In this case, going with Google’s “Recommendations AI” could be a great tool to help you serve up hyper-personalized product recommendations to your users and increase conversions.
Once you have a clear performance goal for your AI, it’s also helpful to assess whether you have the necessary resources in place to bring it to life. There are two foundational areas to consider here: people and process.
When it comes to people, you’ll need to assemble the right team and skill sets to fully unlock the potential of your AI. Highly skilled machine learning engineers and data scientists are critical for optimizing its performance over time.
When evaluating your processes, you’ll want to assess what systems and operations you have in place to support the AI and where the gaps might be. For example, you’ll need a workflow on how to move from design to production, and how you’ll approach testing and continuous improvement of your model. Accenture estimates that 80-85% of all AI projects are stuck in the proof-of-concept stage due to a lack of defined processes or owners with accountability to move things forward.
You’ll also need a process and clear system for collecting data. The potential of your AI is directly related to the quality of the data you have available to use as inputs. If your data is bad, no amount of investment in machine learning will be useful. Make sure you have a plan to capture upstream data like behavior patterns and user profile demographics to feed your future AI. This graphic outlines the levels of data collection you should think about before you start building any tools.

2. Use smart tools to enhance customer experience
Keeping superior customer experience at the forefront of your AI experiments is a great way to ensure meaningful results for your business.
For eCommerce businesses, one tool we find particularly effective is Google’s “Recommendations AI”. This tool is designed to deliver highly personalized product recommendations at scale across all your digital touch points. A major benefit of Recommendations AI is that it draws on Google’s years of experience delivering content recommendations across media properties like Google ads, Google search, and YouTube. It uses dynamic machine learning that adapts to real-time changes in user behavior as well as your product inventory, making it possible to deliver personalized recommendations to your target audience.
A great example of the potential of this tool is demonstrated by IKEA’s success with it. After experiencing a surge in pandemic-driven site traffic and activity, the company wanted to gain a better understanding of its online shoppers, and improve how customers could connect with the digital product catalog. The team implemented Recommendations AI with the goal of improving real-time personalized recommendations and ultimately increasing revenue. After implementing the tool, IKEA saw a whopping 400% increase in relevant product recommendations, a 30% increase in click-through rates, and a 2% increase in average order value.
Whether you’re a small business operating a digital shop, or an eCommerce giant like IKEA, improving customer experience and revenue using a tool like Recommendation AI is very doable.
Thanks to Google’s out-of-the-box approach, you can get the tool up and running in a mere few days, quickly moving from experimentation to deployment at scale. Since it doesn’t require a huge up-front investment in building infrastructure from scratch, it’s an ideal tool for plugging into a composable tech ecosystem where you want to run experiments quickly and learn over time.
3. Take a holistic approach
Implementing out-of-the-box tools like Recommendations AI on their own can yield results, but by introducing AI and machine learning tools within a MACH-enabled tech ecosystem, you’re setting yourself up to unlock the full potential of these tools.
If you’re not familiar with MACH, it’s an approach to web development grounded in four key principles: microservices, API-first, cloud-native, and headless technologies. By embracing this holistic approach, you’re empowering your AI to work as smoothly as possible and deliver game-changing results for your business. Let’s look at an example of this in action.
One of our clients, a data and intelligence company, came to us with a problem: 10% of their customers were returning their product. When customers approached the company for a return, instead of helping people resolve their issues, the company’s automated messaging tried to upsell them, creating more frustration.
The team needed a way to improve their targeted messaging and serve the right message to the right people at the right time. Using Vertex AI, our team built a model to predict which customers were most likely to return the device. It was a success – the AI predicted with 97% accuracy which customers were likely to return the product. As a result, the team crafted personalized messaging designed to provide product information and guide customers to next steps.
If not for the flexibility of an existing MACH environment, building, and deploying this model to arrive at actionable insights would take a lot longer. Thanks to the API-first nature of MACH, data can easily flow between all your tools. Simple integrations help you set your AI up for success, similar to the setup of your other business operations. Since your front-end and back-end environments are decoupled, there are fewer dependencies and less risk overall when it comes to making updates to how your AI runs. All of these are benefits that come from embracing a MACH ecosystem, helping you move swiftly from ideation to monetization, and drastically reducing the time to market for your AI or machine learning tool.
Ultimately, if you are looking to launch or expand your current AI capabilities, getting clear on your business goals, selecting smart tools to enhance customer experience, and embracing a MACH ecosystem to evolve your technology will lay the essential groundwork for long-term success.
If all of this sounds overwhelming, it doesn’t have to be. At Apply Digital, we work directly with clients who want to advance their digital strategies through machine learning and AI, helping them build custom solutions from the ground up. If you would like to explore how we can support you, reach out to us at hello@applydigital.com. We’re happy to help.
Partner with us
Together, we can deliver innovative solutions and drive your digital change journey.
Keep learning
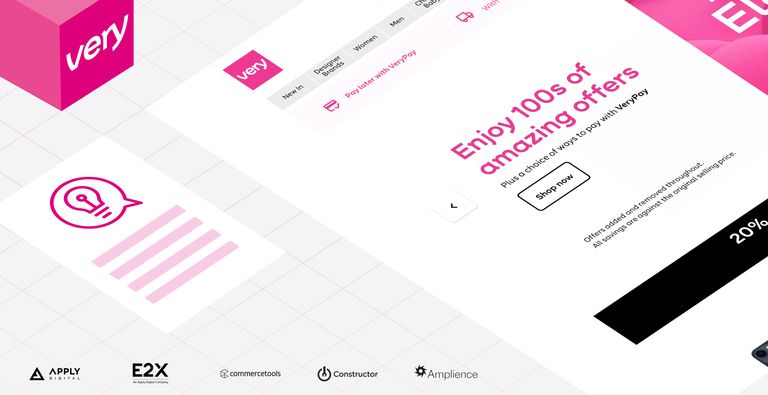
6 Tips from a Global Retailer’s Digital Transformation
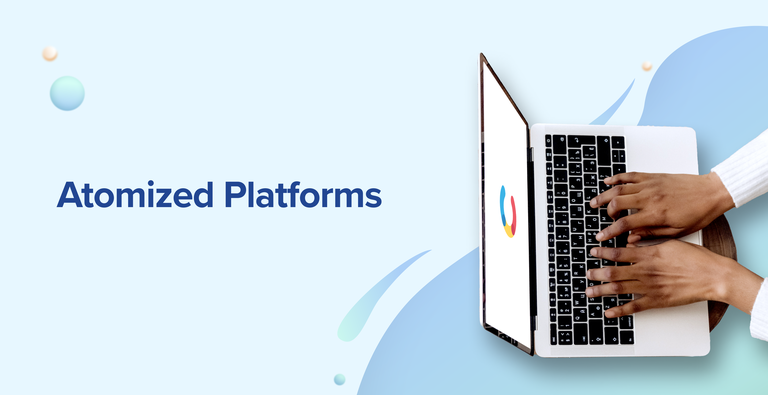